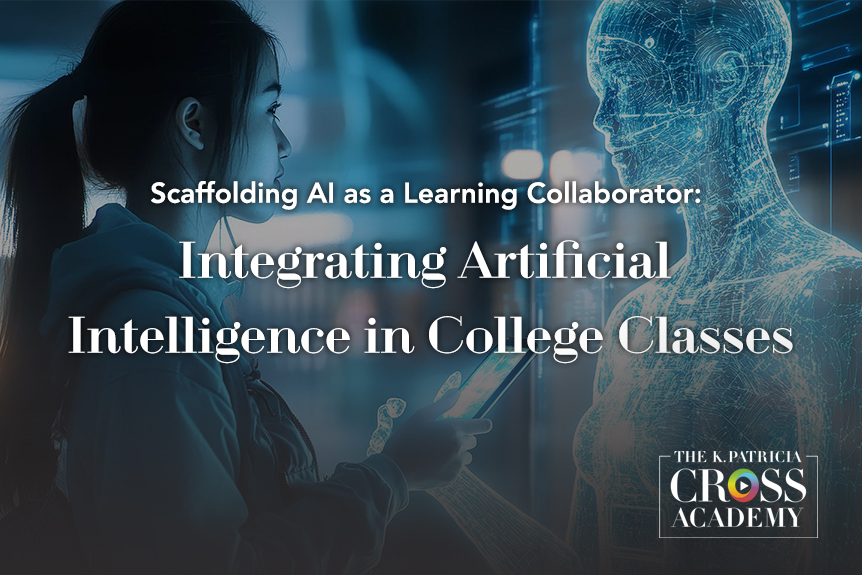
Introduction
The integration of Artificial Intelligence (AI) in higher education represents more than technological advancement—it signals a pedagogical and cultural transformation (Ouyang & Jiao, 2021). While universities actively implement AI platforms and train faculty, a critical gap persists: students often lack structured guidance in AI engagement and integration into their learning process. This delayed exposure to rapidly evolving AI tools creates tension between empowering learners and potentially making them passive recipients of information (Dhawan & Batra, 2020). Recent research suggests that while Generative AI can enhance metacognitive skills and communication, maintaining student autonomy remains vital (Yan, Nakajima, & Sawada, 2024). As the U.S. Department of Education’s 2023 report emphasizes, AI should function “more like an electric bike and less like robot vacuums,” with humans directing the decision-making process.
Of course, neither students nor instructor can learn all that AI has to offer overnight. This article provides educators with a framework for integrating AI into their classrooms, acknowledging that mastering AI capabilities requires time and structured guidance – and that it cannot merely be extra-curricular learning but, instead, is best embedded into all disciplines. This article guides instructors in fostering students’ AI literacy, enabling them to maintain authority over AI, critically assess its capabilities and limitations, use it to advance real-world solutions, and evaluate its appropriate applications.
Building a Foundation: Students as AI Drivers
Successful AI integration depends on framing AI as an intelligent collaborator rather than a simple answer generator. Students must develop a nuanced understanding of AI’s capabilities and limitations through instruction matched to their experience level (Speicher, n.d.).
The goal is to position students as active drivers of AI interactions rather than passive consumers of AI-generated content.
To get started, consider having students collaborate with AI to complete the following brief introductory activities:
- Practice AI Prompt Engineering: Using your institution’s approved AI platforms, guide students through a progression of prompt development. Start with basic prompts like “Tell me about climate change,” which typically yield broad, unfocused responses. Progress to intermediate prompts such as “Explain the three main causes of climate change and their impacts,” which provide more structure. Finally, introduce expert-level prompts: “As an environmental science educator, explain the three main causes of climate change and their impacts. For each cause: describe the mechanism, provide one specific example, and include relevant statistics. Format the response in clear sections with headers.” This advanced prompt demonstrates the key elements of effective AI communication: establishing context, defining specific requirements, and requesting structured output.
- Develop Interactive Dialogue Skills: Encourage students to engage in meaningful exchanges with AI. For example, in an education policy course, students might begin with a complex query like “How does the implementation of a new educational policy impact student engagement in marginalized communities?” AI often offers to elaborate on specific points, where students should practice following these threads through targeted follow-up questions. These interactions should then fuel small group discussions where students analyze and compare their findings with peers.
Creating a “safe to fail” learning environment is foundational for these activities. Students need space to experiment with AI without fear of making mistakes
In addition, this safe environment can promote critical peer-to-peer conversations that are needed for real-world applications, too! This supportive atmosphere encourages both content exploration and technological experimentation, helping students navigate the often-overwhelming landscape of rapidly evolving AI capabilities.
Fostering Critical Awareness
As students become proficient with AI communication, they may rely too heavily on AI-generated content. Beyond simply warning against this as overstepping academic integrity and possibly plagiarism, educators must help students understand how excessive AI dependence can perpetuate biases and compromise academic integrity. This section outlines strategies for developing students’ critical analysis skills to avoid over-reliance on AI.
- Analyzing AI Limitations: While AI systems continuously improve through updates and user feedback, they remain imperfect tools designed by humans. To demonstrate these limitations, have students analyze AI-generated summaries of course readings. Direct students to compare these summaries against the original texts, identifying both accurate representations and significant omissions. Through small and large group discussions, explore how factors such as algorithmic bias, prompt construction, and training data limitations influence AI outputs. This exercise helps students develop a more nuanced understanding of AI’s capabilities and constraints.
- Developing Ethical Framework: Students must learn to use AI as a collaborative tool while maintaining mandated academic integrity. However, this from-the-top authority may not be enough for students to fully understand the ethics of using AI. Therefore, emphasize the importance of validating AI suggestions against scholarly sources and properly citing original research rather than AI outputs. Guide students in developing their own analytical perspectives rather than simply accepting AI-generated conclusions. Engage students in discussions about critical ethical considerations, including:
- Bias and equity in AI systems
- Data privacy and security concerns
- The risk of skill atrophy through over-reliance
- Transparency in AI decision-making
- Environmental costs of AI infrastructure
These discussions strengthen students’ data literacy while teaching them to harness AI’s potential ethically and effectively. By integrating these principles into course content, students learn to leverage AI as a learning tool while maintaining academic rigor and independent thinking.
Advancing to Real-World Applications
Within this paper’s suggested framework, students progress through distinct stages in their AI learning journey. First, they experiment in a safe environment, exploring AI’s various applications. Next, they develop critical reflection skills to evaluate AI’s appropriate uses and limitations. Through this progression, students learn to position themselves as thoughtful drivers of AI collaboration rather than passive users.
Once students understand their critical role in assessing information provided by AI (and their peers), they can move forward to engage critically with peers and colleagues, assessing the quality and logic of all inputs – both human and artificial.
Consider the following AI and peer collaborations to advance AI usage into real-world considerations:
- Problem-Based Learning with AI: Present students with local community challenges that require innovative solutions. Guide them in using AI to analyze relevant datasets and develop potential interventions. For example, students might use AI to examine neighborhood food desert data and propose evidence-based solutions. This process should include careful consideration of the ethical implications of their AI-assisted analysis, ensuring that proposed solutions remain grounded in human understanding of community needs.
- AI-Enhanced Project Development: Encourage students to strategically incorporate AI into their summative projects as an analytical and organizational assistant. Students should identify specific areas where AI can enhance their work without compromising originality. For instance, AI might help structure research findings or generate preliminary outlines, while students maintain responsibility for critical analysis and creative synthesis.
As students master these advanced applications, they demonstrate their ability to leverage AI while maintaining intellectual autonomy. This progression from basic skills to sophisticated implementation creates a foundation for evaluation of AI usage. The next section explores how instructors can collaborate with students to assess and refine their AI engagement, ensuring that students develop lasting skills for ethical and effective AI utilization in their academic and professional futures.
Assessment Strategies
Lastly, once comfortable with AI integration, consider the following comprehensive evaluation methods that encourage student self-reflection and ongoing skill development.
- Process Documentation: From initial prompts to iterative refinements, have students practice reflecting and documenting their AI communication and collaboration. This documentation demonstrates the evolution of their prompt engineering skills and reveals patterns in their AI usage and decision-making. Instructors can integrate these records into both formative assessments and summative evaluations, perhaps through a digital journal or structured log where students regularly reflect on their AI interactions and learning outcomes.
- Collaborative AI Assessment: Collaborate with students to create meaningful evaluation criteria for AI usage. This partnership could examine students’ sophistication of AI engagement, improvement in interaction strategies, and the balance between AI assistance and human oversight. Through this collaborative assessment process, both instructors and students gain deeper insights into AI’s capabilities and limitations. Students learn to critically evaluate AI outputs while instructors gain perspective on effective integration strategies. This approach transforms traditional assessment into a shared exploration of AI’s role in learning.
These evaluation methods serve to prepare students for continued ethical and effective AI use beyond the classroom, fostering skills that transfer to future academic and professional contexts. Through careful documentation and collaborative assessment, students develop lasting habits of critical engagement with AI technology.
This framework offers educators a practical roadmap for meaningful AI integration in higher education classes. Through carefully sequenced activities and assessments, instructors can guide students from basic AI literacy to sophisticated application, fostering technical competence, critical thinking, and reflective collaboration skills. By following these strategies, educators empower students to become independent AI users who maintain their intellectual autonomy while leveraging AI’s capabilities effectively.
Below are some strategies from the K. Patricia Cross Academy Library to continue engaging students in the metacognitive process needed to be reflective AI-users and contributing citizens in social and learning communities:
Potential Techniques:
Potential Crosscurrents Blogs:
Suggested Citation
Barkley, E.F., & Major, C.H.Gutenson, L. D., & Morris, S. J. (n.d.) . Scaffolding AI as a learning collaborator: Integrating artificial intelligence in college classes. CrossCurrents. https://kpcrossacademy.ua.edu/scaffolding-ai-as-a-learning-collaborator-integrating-artificial-intelligence-in-college-classes/
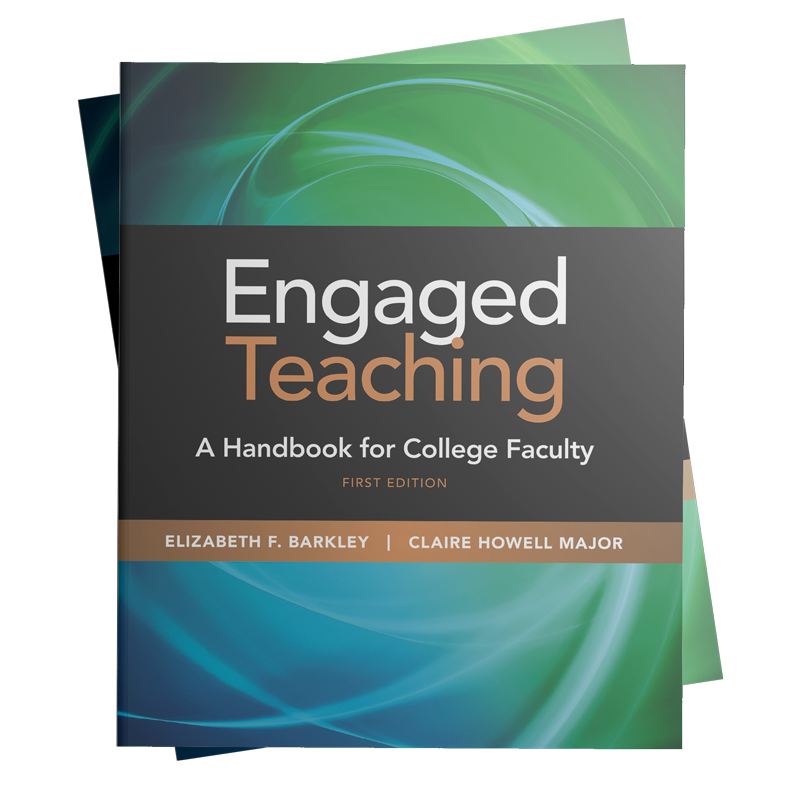
Engaged Teaching
A Handbook for College Faculty
Available now, Engaged Teaching: A Handbook for College Faculty provides college faculty with a dynamic model of what it means to be an engaged teacher and offers practical strategies and techniques for putting the model into practice.