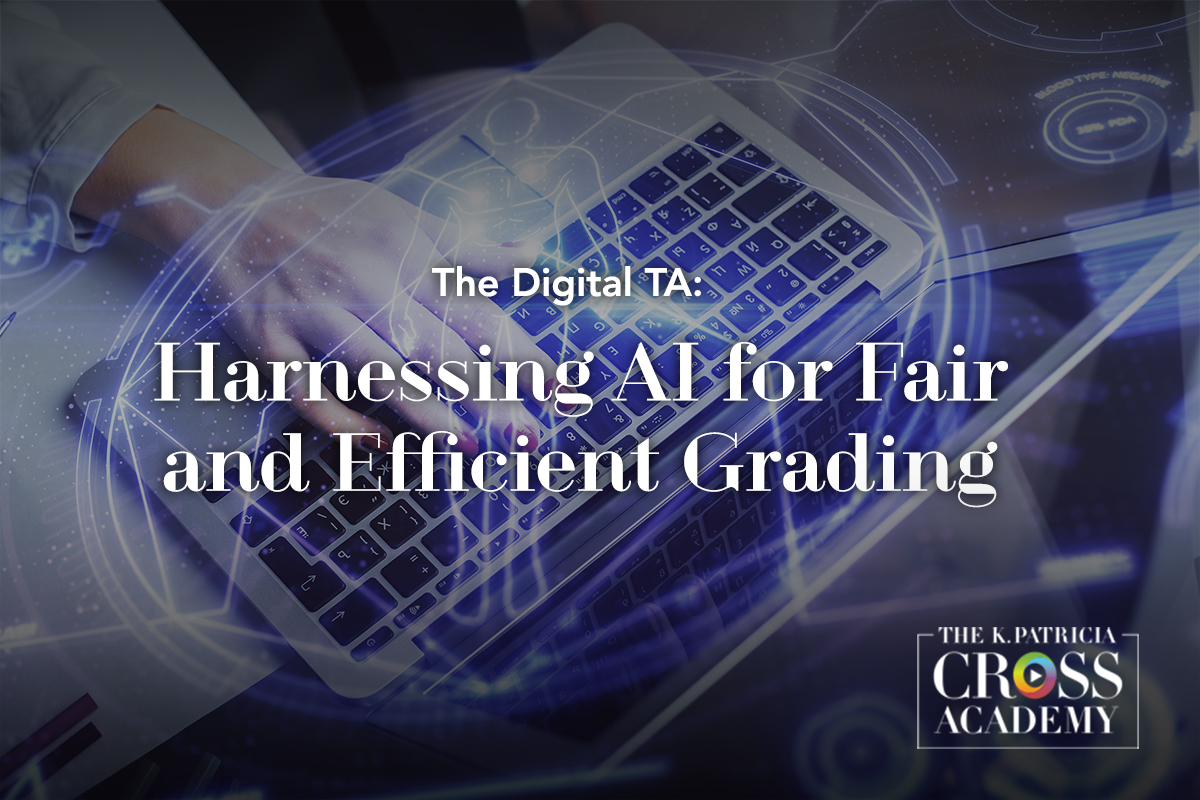
Introduction
The advent of Large Language Models (LLMs), such as ChatGPT, Claude AI, DeepSeek, and Google Gemini, has rapidly transformed higher education. From crafting syllabi to generating lesson plans, many educators are leveraging these Artificial Intelligence (AI) tools to enhance the effectiveness of their teaching practices. Among the most intriguing applications of this emerging technology is its potential use in academic grading, offering opportunities to improve efficiency and transparency. While some instructors and students view this development as largely positive (Calamas, 2024), others have raised concerns regarding the ethics and quality of feedback provided by LLMs (Kelly, 2024; Taylor, 2024). This article takes a nuanced approach to the topic, examining how AI can serve as a complementary tool to support, rather than replace, human-led educational assessment.
The Promise of AI in Grading
AI has the potential to greatly assist human graders across disciplines. Liu et al. (2024) highlight that AI can save mathematics instructors time by handling repetitive grading tasks and applying rubrics consistently, possibly reducing human bias. Similarly, Calamas (2024) found that Gradescope, an AI-assisted grading tool, significantly cut grading time in an undergraduate engineering course while providing detailed feedback on problem-solving steps. This feedback helped students understand their mistakes and how points were allocated. Furthermore, 84.62% of surveyed students perceived anonymized, rubric-based grading as fairer, ideally fostering trust and reducing bias. AI tools like Gradescope demonstrate their potential to reshape traditional grading practices by addressing these persistent challenges.
AI can also assist with grading essays and short-answer questions by evaluating structure, spelling, and grammar, allowing instructors to focus on the less formulaic aspects of writing, such as “content richness, vocabulary use, and overall quality” (Almegren et al., 2024). Jonäll (2024) adds that AI can provide “specific, useful, and appreciated feedback,” most effectively in tasks requiring straightforward evaluation. These applications suggest that AI is not only transforming how we grade but also opening the door to more meaningful interactions between educators and students.
Current Limitations of AI in Grading
Despite these exciting possibilities, using AI in grading poses significant challenges for instructors. While using AI tools may enhance grading consistency through rubric-based assessments, LLMs struggle with providing complex, nuanced responses to both written work and mathematical problem solving. Because they are limited in capturing contextual subtleties, they cannot fully assess the creativity, originality, and deep insights of student work (Almegren et al., 2024; Calamas, 2024; Jonäll, 2024; Liu et al., 2024). If instructors rely solely on these tools for responding to assignments, they risk delivering feedback that feels prescribed and disingenuous.
Using AI as an assessment tool also raises ethical concerns regarding accuracy, student privacy, intellectual property rights, and algorithmic bias (Calamas, 2024; Jonäll, 2024; Li et al., 2024; Taylor, 2024). Perhaps most importantly, AI cannot replicate the integrity of instructor presence. These limitations remind us that the heart of effective assessment lies in human judgment and connection.
As we explore strategies for integrating AI grading practices responsibly, the focus must remain on enhancing—not diminishing—the depth and authenticity of feedback.
Responsible AI Grading Integration Strategies
When considering how to use AI responsibly to provide feedback on assignments, centering meaningful human judgment is paramount. Echoing the recommendations of Almegren et al. (2024) and Jonäll (2024), we suggest taking a hybrid approach to using AI in grading, where the strengths of artificial intelligence and human educators create an efficient and effective grading process.
It’s important to recognize that developing this process takes time and intentional collaboration, requiring educators to critically engage with AI tools, experiment with different workflows, and continuously refine their approach to ensure that technology enhances rather than replaces nuanced academic assessment.
Below is a list of feedback best practices for balancing AI-generated insights with thoughtful, personalized feedback from instructors:
-
Prioritize Student Privacy
- Protect student data by using institution-approved AI tools. Treat LMS platforms as digital public spaces and avoid sharing confidential, student-identifying information (“Navigating Data Privacy,” n.d.). As Heikkilä (2023) notes, once data is added to an LMS, it is difficult or impossible to remove it from the AI system. When in doubt, consult your institution’s privacy policies.
- Be sure to highlight the steps you will take to protect student privacy and provide alternatives if students object (“Practical Strategies for Teaching with AI,” n.d.).
-
Humanize Feedback
- Maintain transparency with students regarding the use of AI in grading by notifying them in advance of its use. Context is key. Explain when and how AI will be used in your course to assess their work (“Practical Strategies for Teaching with AI,” n.d.).
- Use the student’s name and reference specific details about their submission to show personal engagement. Strive for a conversational tone to make feedback feel approachable.
- Below is an example that illustrates the nuanced difference between a standard AI draft response and a carefully tailored, student-specific piece of feedback
- AI-Generated Draft Response: “Your thesis is clear and aligns with the rubric criteria. Consider adding more evidence to support your argument in paragraph three.”
- Instructor’s personalized Response: “You’ve chosen a strong thesis, Emma, and your analysis in paragraph two is particularly compelling. Adding evidence in paragraph three would make your argument even stronger—perhaps by referencing XYZ from the course material.”
- Below is an example that illustrates the nuanced difference between a standard AI draft response and a carefully tailored, student-specific piece of feedback
-
Balance Clarity and Depth
- Combine AI generated bullet points for clarity with complete sentences for context and personalization. Avoid overwhelming students with overly lengthy or fragmented feedback—focus on actionable and prioritized comments (Covington, 2024).
-
Consider Cognitive Load:
- Organize feedback logically (e.g., strengths, areas for improvement, actionable suggestions). Use accessible language and format feedback to minimize cognitive overload (Covington, 2024).
-
Maximize Efficiency Without Sacrificing Quality
- Set clear parameters for the AI’s role to prevent over-reliance or redundancy. Use templates or pre-set AI prompts aligned with rubrics for efficiency while leaving space for individualized instructor input. This approach is particularly effective for assessments where specific elements can be standardized.
-
Continuously Reflect and Improve
- Regularly audit AI systems for accuracy and fairness, as AI tools may “hallucinate” and produce false information.
- The training models AI uses to create output may reflect harmful stereotypes that perpetuate biases. To address these challenges, be sure to carefully read all feedback on student work provided by AI before sharing it with students (“Practical Strategies for Teaching with AI,” n.d.).
- Foster a learning environment where students feel comfortable voicing concern about any AI-generated feedback they receive (“Practical Strategies for Teaching with AI,” n.d.).
- Regularly audit AI systems for accuracy and fairness, as AI tools may “hallucinate” and produce false information.
Conclusion
The integration of AI into academic assessment is not about replacement, but enhancement. By thoughtfully balancing technological capabilities with human insight, educators can transform grading from a mechanical, time-consuming task into a meaningful dialogue that supports student growth.
As we navigate this emerging landscape, our north star must remain the core purpose of education: fostering genuine learning, understanding, and human connection.
Below are some additional blog posts from the K. Patricia Cross Academy CrossCurrents Library that may provide further guidance as you continue to consider how you will incorporate AI into your college classroom:
Discover how ChatGPT is transforming college education by fostering critical thinking and creativity while sparking essential conversations about its ethical challenges, like plagiarism and over-reliance. This thought-provoking blog post invites educators to weigh its transformative potential against its complexities in shaping the future of learning.
Explore how artificial intelligence can act as a dynamic collaborator in college classrooms, helping students tackle complex problems, foster creativity, and build critical thinking skills. This insightful blog post also delves into strategies for integrating AI ethically and effectively, preparing learners for a future shaped by advanced technology
Suggested Citation
Barkley, E.F., & Major, C.H.Gutenson, L. D., & Morris, S. J. (n.d.) . The digital TA: Harnessing AI for fair and efficient grading. CrossCurrents. https://kpcrossacademy.ua.edu/the-digital-ta-harnessing-ai-for-fair-and-efficient-grading/
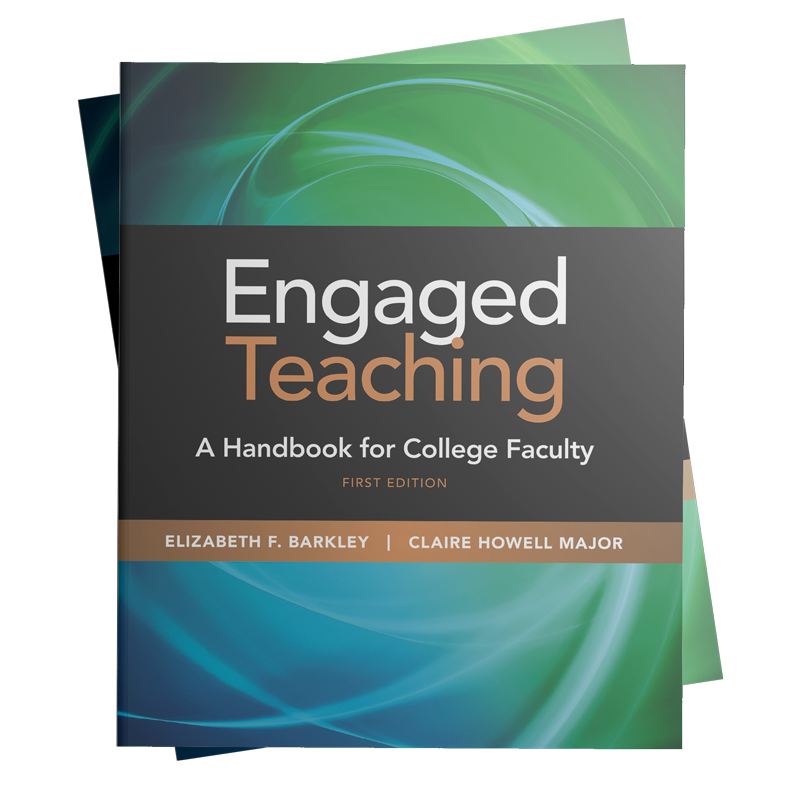
Engaged Teaching
A Handbook for College Faculty
Available now, Engaged Teaching: A Handbook for College Faculty provides college faculty with a dynamic model of what it means to be an engaged teacher and offers practical strategies and techniques for putting the model into practice.